#5 Cycorp: Dr.Lenat takes us through his decades of experience in AI and his recent work in Knowledge Representation and Reasoning
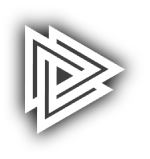
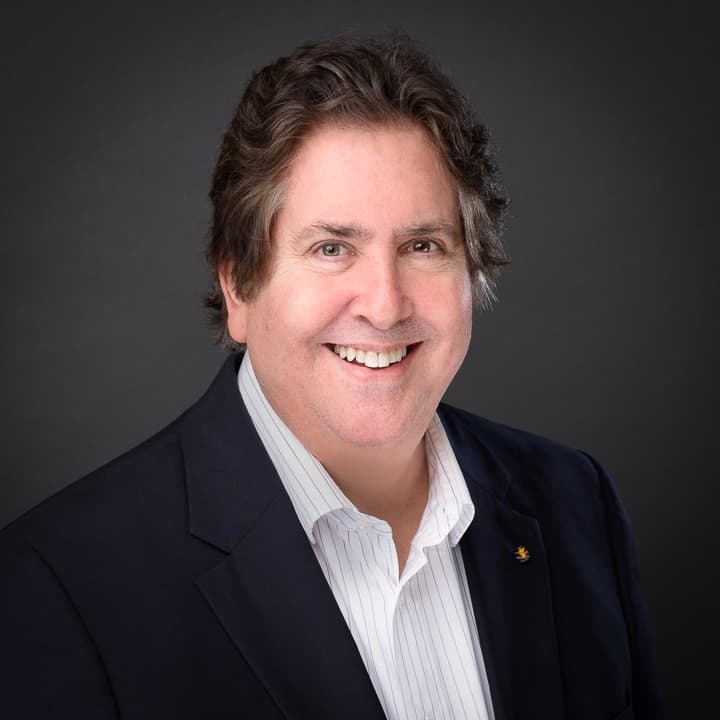
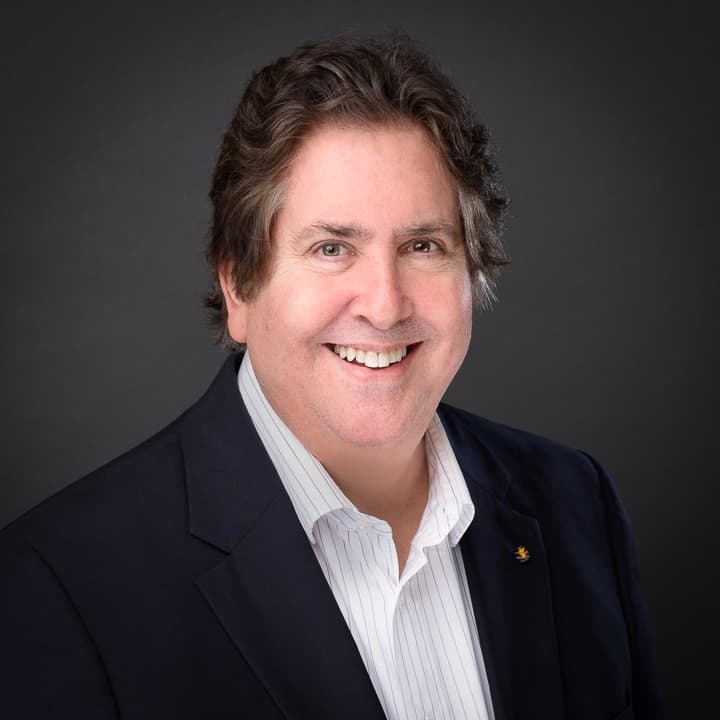
#5 Cycorp: Dr.Lenat takes us through his decades of experience in AI and his recent work in Knowledge Representation and Reasoning
SUBSCRIBE ON:
Introduction To Guest
Douglas B. Leonard is one of the world’s leading computer scientists. He’s the founder and CEO of Cycorp, a knowledge, representation, and reasoning software company. He has been a professor of computer science at both Carnegie Mellon and Stanford University, and has received numerous honors. Dr. Leonard was awarded the biannual I JCI Computers and Thoughts Award, which is the highest honor in Artificial Intelligence. Dr. Leonard has authored over 100 publications in the area of machine learning, automatic programed synthesis and knowledge based systems. He has served on the scientific advisory board of both Microsoft and Apple. Dr. Leonard has also received an undergraduate degree from University of Pennsylvania.
The Call To Action
Dr. Leonard learned to program computers in the 1960’s when he was in high school, this was during the era when mainframe computers began to emerge. Dr. Leonard was looking at all of the problems facing the world, really the same problems we are witnessing today: poverty, hunger, disease and so on. With a drive to make a difference, he realized that in order to do so, we need to be smarter as a species.
With this realization, and an interest in computers and technology, Dr. Leonard started to learn more about Artificial Intelligence. Through his learnings and during his years as a professor, Dr. Leonard discovered that AI held the potential to assist in making his dreams a reality — by amplifying human brain power. In turn, making us smarter as both humans and as a species to improve the world as a whole. Throughout the 1970’s and into the early 80’s, Dr. Leonard studied and worked with AI — specifically with machine learning and natural language understanding. However, he and his colleagues kept hitting a brick wall in their research. They built AI systems that looked promising to start with — with the veneer of intelligence and the veneer of understanding, but nothing that would scale. What they realized was missing was common sense rules and accurate judgements about how the world works: causes precede effects. At the time, to get computers to reason through logic was extremely slow, very impoverished, and quite expensive. Dr. Leonard’s second realization was that to properly capture all of this common sense knowledge, it would require thousands of years of effort.
It was not until a once in a lifetime opportunity presented itself where Dr. Leonard’s life completely changed. This opportunity consisted of leaving Stanford University and moving to Austin, Texas to work hand in hand with Bob Inman, one of the smartest people Dr. Leonard has ever met. Dr. Leonard was offered the role as the lead scientist at the first research consortium.
Coming Up With The Idea
Working as the principal scientist at the consortium, Dr. Leonard began to codify tens of millions of things we know about the world – not the facts, nor the things that are findable via Google – but the basic and/or common sense things that nobody says or writes down. For example, water flows downhill or the sun appears to be yellow. There was a motivation for Dr. Leonard and his team to make us all smarter by codifying human common sense, to serve as a foundation for Artificial Intelligence that would not be brittle the way that it was in the 1970’s, or quite frankly, the way it still is today.
During the time between 1984 and 1994, Dr. Leonard kept his title at the principal scientist for the MSNBC Consortium, and later spun out as a separate company Cycorp by the end of 1994. He is proud to say that Cycorp has been operating profitably for the last 25 years, ever since the founding of the company. Dr. Leonard explains how during the 1960’s and 1970’s, AI researchers (which included himself) have built a large number of knowledge based systems. And all while doing so, the logicians of the world were refining adequately expressive, logical formalisms to capture complicated human expressions. They could not crack this common sense, knowledge, representation and reasoning through an engineering approach, but rather through a scientific research approach. So day by day, month by month, year by year, decade by decade, Cycorp uncovered about 150 significant problems, or “learning experiences”, all while revisiting their thinking and foundation of work when they were proven wrong.
Testing The Product
Cycorp created the revolutionary AI platform called Cyc. This platform consists of human reasoning, knowledge, and logic at enterprise scale, all based on collecting explicit logical descriptions of common-sense knowledge.
From founding Cycorop in 1994, Dr. Leonard and his team had to come up with ways of replacing the reliance on the assumption of global consistency, with the knowledge base and the rule based with something else. They replaced global consistency with the notion of local consistency. For example: we know, intellectually, that the surface of the earth is roughly spherical. But we live our everyday lives as though the earth’s surface is flat. This tends to work out OK, because it is locally flat and we hardly ever think about the curvature of the Earth. If you’re in the US but talking to someone in Australia, you’d never think about the fact that that person is actually oriented upside down to you. Dr. Leonard and his team could produced the knowledge base because it was divided up into little regions, contexts or micro theories, which was locally consistent.
Validating The Market
Cycorp’s potential market is really everything that software is being used for today. They can augment almost anything that has even a small component of human common sense. Anything where knowledge, understanding, and explanation would really help humans who are performing the task. This has not been an easy feat, especially since 30 years ago, or even 10 years ago, most people didn’t realize that they needed something like this type of Artificial Intelligence. Dr. Leonard explains how validating the market has gotten easier in the last decade, mostly because of the resurgence of what people currently call AI. However, when people mention AI today, they usually mean statistical machine learning or training multilayer neural networks on big data. Like Siri or Deep Mind for Google.
However, if you have an application in healthcare, finances, or the military, the use of Artificial Intelligence can be a life or death decision. People want explanations, and rely on the ability for software to fall back on general common sense and knowledge. At Cycorp, they’re trying to create an awareness in this marketplace, along with a way to fulfill this need. Cycorp is trying to stay away from problems which are too easy because these problems can be solved by statistical machine learning. Yet Cycorp is also trying to steer away from problems that are too hard as well. Cycorp focuses on problems in the middle of these two extremes, problems where the best experts are much, much better than the average practitioners who are solving that particular problem for a living. For instance, the cutting edge of complicated medical diagnosis or being able to look at complicated supply chains and diagnose interdependencies that weren’t obvious in the beginning.
At Cycorp, they are looking for problems that don’t just require an answer, but where it would be helpful to see the top two, three, or four answers, along with the pro and con arguments giving our step by step lines of reasoning for each one. Cycorp focuses on health care and energy because without their product, in order to build a solution from scratch, it might take decades and hundreds and millions of dollars. If you do have Cyc, along with a vertical customized product that you just have to customize further, it would take weeks or months, and cost maybe hundreds of thousands of dollars. Now they are down in the range where companies see the Cycorp product as wildly cost effective. Cycorp can actually gain commercial business this way.
Company Funding
Over the last three decades since the birth of Cycorp, Dr. Leonard made an intentional decision to keep their corporate revenue down to about five or six million dollars a year. The reason being was Dr. Leonard and his team did not want to be burdened with the curse of having to make decisions prematurely when they knew they had many learning experiences in their future. He knew that if they had too much money, Dr. Leonard would have to make decisions that they’d have to live with forever, and because of the nature of his work, half (or more) of those decisions would be wrong. In many cases, Dr. Leonard is more than grateful for this monetary decision.
Finding A Team
For Dr. Leonard finding a team at Cycorp has been an interesting journey. With a degree in computer science, he initially assumed that the best people to hire would be computer scientists, which they indeed hired. However, over the course of the first year or two, he quickly realized that the talents really needed were skills different than the ones taught in computer science classes. Dr. Leonard was looking for people who were not paradigm locked to think in terms of data science and data analytics. To this day, Introspection thinking is key. The talent needed required a “different” type of team member. Employees who proved to be an asset were people who could look at a sentence, for example, that had an ambiguous word or pronoun, introspect, and think of a solution in a more creative fashion. Within a couple of years, Cycorp replaced all of the computer scientists with philosophers, engineers, and other people who were trained in facets other than computer science.
He reiterates that if they had too much investment money, Cycorp wouldn’t’ have been able to reverse their initial decision on hiring.
Challenges Encountered
While working on developing basic reasoning, Dr. Leonard points out that the interstices between basic reasoning principles would allow for small discrepancies to creep in. For example, things could be true for an instance, at one level of granularity. This computer is a solid object, but at an atomic level, it’s mostly empty space, etc. Secondly, there are technological challenges and needing more expressive logic, not just three words of logic, but perhaps 10-20 sentences of in depth logic.
The other technological challenge that Cycorp faced was to generate understandable English paraphrasing from an underlying logical representation. This was so users can understand what the system was doing and saying, while following step by step reasoning. Luckily, Cycorp’s first attempts were successful in the case of logic. In the case of natural language generation, Cycorp took a compositional approach. Each relation had a way of saying that relation, and each argument had a way of saying its argument. You generated stilted sentences, but they were still understandable.
The fourth and final example of a challenge Cycorp overcame was getting the system to reason quickly. The breakthrough they had was to separate the epistemological problem, i.e.: “what does the system know”, to “how can the system reason effectively.” As soon as you separate the two, Cycorp found that you can have two different representation languages. You can use logic as a nice, clean way of representing what the system knows and then you can have a heuristic level, special purpose data structure to efficiently (and redundantly) represent some of the information so you can answer specific questions really, really quickly.
Future Endeavors
In the future, Cycorp hopes for AI to augment human brain power, in much of the same way that electricity and electrification, augmented and amplified human muscle power. Now we can travel faster than our legs could carry, communicate further than we can shout, wash dishes and cut lawns faster and better. In the same way, Cycorp believes that Artificial Intelligence can and will augment and amplify human brain power so we can think of solutions to harder problems faster than ever before.
Humans have a left and right brain hemisphere. The right brain hemisphere handles emotions, forming patterns and recognizing patterns, similar to neural network machine learning systems. Our left brain hemisphere handles logic and deliberate thinking. Dr. Leonard imagines future AI similarly. The right brain will be the machine learning half, and the left brain the psychological-like half. The machine learning side will propose hypotheses statistically, while the left brain half will sit back, think, and decide which ones not only make the most sense, but why and how could they test out some of those hypotheses and then go back to the right brain to confirm or disconfirm with empirical data.
In the future, Cycorp believes this back and forth hybrid reasoning is what’s going to power the AI applianess, helping us become smarter as individual human beings and as a species. It will help us tackle some hard problems, not just from a business standpoint, but from a societal viewpoint, which includes many of the difficult problems facing the world today.
Advice for Key Industry Leaders
Zone in on cost saving and maximization of profit. Most people are too focused on using right-brain, machine learning, type-A eyes exclusively because it’s a cheap option. However, when there is an unexpected change in your system, this narrow minded thinking will only harm you in the long run. For example, if a pandemic occurs or there is a political change that cuts off some supplier in your supply chain, to name a few. Your business has to be able to rapidly adapt and be resilient. Start building flexibility and resiliency in your business now so you’re prepared as the world changes. Stay long-term focused and devote more energy into building high resilience and agility.
Advice for Aspiring Entrepreneurs
Dr. Leonard’s advice for aspiring entrepreneurs is try and keep an open mind in terms of your staffing. Do not just hire people who have trained in data analytics, but think about bringing on employees with degrees in philosophy, linguistics, and engineering. Those who are trained to introspect and think outside the box. The second piece of advice is to partner with organizations that are working on slightly different types of applications than your company. This way, you will have more complete and systematic organic solutions. Forming these unique partnerships will make a huge difference in providing solutions that are going to be attractive and useful for long term customers.
www.cyc.com
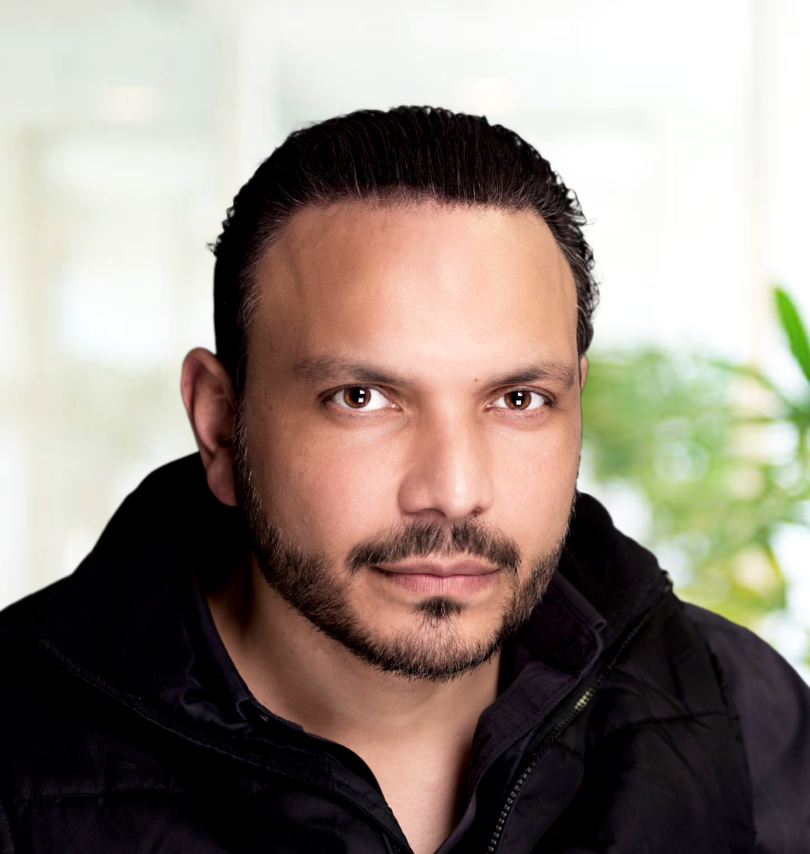
About the Host
Ari Yacobi is a data scientist, a teacher and a storyteller who has spent his career at…Read the Bio