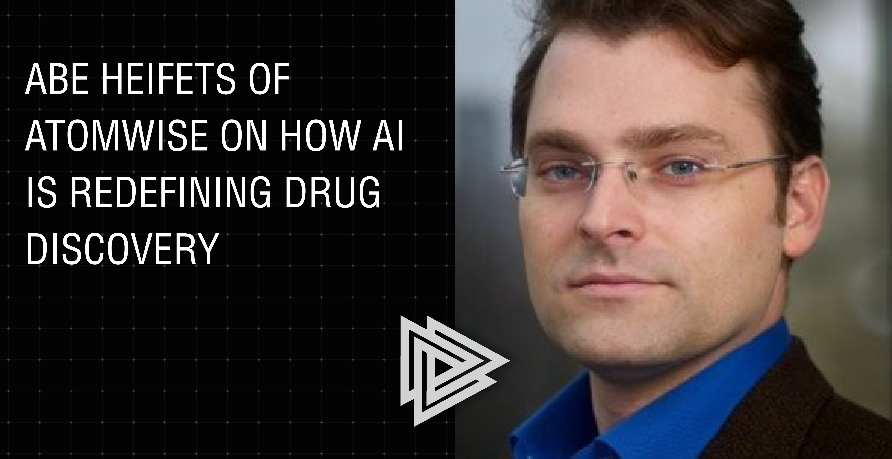
SUBSCRIBE ON:
Introduction to Guest
Dr. Abraham Heifets is CEO and Co-Founder of Atomwise, where he and Co-Founder Dr. Izhar Wallach invented the use of deep convolutional neural networks for drug discovery. Dr. Heifets was a Massey Fellow at the University of Toronto—a center for AI innovation—and a Fellow of the Ontario Brain Institute. His doctoral work applied machine learning and classical AI techniques to organic synthesis planning, which is a long-standing challenge in chemistry. His vision of AI bringing better, safer and more potent drugs to patients was recently featured in the July 2019 Moonshot issue of Newsweek. Dr. Heifets is a thought-leader on the use of AI for drug discovery and is an author on 24 papers, patents and patent applications. Dr. Heifets has presented his work to the U.S. GAO, the National Institutes of Health, the American Chemical Society, and the Association for the Advancement of Artificial Intelligence.
Abe’s degrees are all in computer science – think good old fashion Artificial Intelligence. Abe wound up working for IBM Research in Boston for about five years working on big data problems. Up until this point, he hadn’t looked into chemistry or biology. What happened was, a good friend of his decided to become a doctor. After Abe’s friend began his pre-med courses, he would complain to Abe about organic chemistry (in particular). To Abe, however, organic chemistry sounded fun, since the algorithms in organic chemistry are very similar to algorithms that computers use. For example – IBM at the time created computers that started to beat humans as chess, which was a huge breakthrough, and one that had been open for 80 years. When it came to the problem of organic chemistry, Abe realized it was deeply linked to the problem of playing chess. Abe then began taking organic chemistry courses at Harvard to see if he could actually do the work. This new venture (or hobby) was how Abe got into the life sciences.
Abe soon decided to take his education further. He had the good fortune to complete his PhD at the University of Toronto where modern machine learning was being discovered. Abe began to shift from old fashioned AI techniques to machine learning techniques, while learning about neural networks and convolutional neural networks. There Abe met his cofounder – where together they combined protein analysis algorithms, big data approaches to chemistry, and machine learning to create Atomwise.
Coming Up with The Idea
Atomwise really began as a research question. People have been trying to use computers for chemistry for decades. The use of modern machine learning of convolutional neural networks of deep learning was new, essentially what Atomwise brought in, but the idea of computers and chemistry was not new. Every major industry uses computers to do its designs – with most of the prototypes made tested in the computer first. Pharma is really the last industry where you build every single prototype manually. So, the idea that we could use computers was not working because the accuracies were never good enough. This in itself sparked an idea in Abe. With computational chemistry literature (datasets), people began looking at the weaknesses of these open problems. Luckily, Abe and his co-founder had access to these new datasets while earning their PhD’s.
Abe and his cofounder started discussing the fact that data sets enabling the use of machine learning algorithms are just being curated and formalized. And maybe, together, those datasets could solve these long-standing problems. Atomwise really began with that open research question, which, essentially, kept working. Even when it was tested, it kept working. Abe knew they were onto something big, and it was worth taking the risk to implement it beyond paper. Creating a product with the drive and notion to have a deep and everlasting impact on the world.
Raising Capital
The company was originally founded in Toronto where Abe’s day to day duties entailed raising funding for Atomwise. This was a discouraging task, as he kept receiving feedback that the product was not going to work. So, in 2015, Abe and his co-founder relocated to the Bay area for Y Combinator. It was there where they met Tim Draper of Draper Fisher Jurvetson, who funded two companies working on a similar idea 20 years ago but decided to take a shot at it again with Atomwise
At this time, Abe wound up giving a very similar presentation to what he had been pitching a couple months earlier in Toronto. However, there was a huge difference in perspective and mentality between Toronto and the Bay Area. People in both places looked at the same data and came to the exact opposite conclusions about whether Atomwise was fundable. At this moment, Abe and his co-founder knew they found their home to grow the company.
Artificial Intelligence for Drug Discovery
Atomwise uses AI to help design drugs. For a drug to work, it has to stick to a disease protein to shut down the functioning of that protein to reset the disease process. That same drug simultaneously has to bounce off proteins in your liver, your kidneys, your heart, or your brain that you want to keep functioning – organs that are important to your health and your happiness. Fundamentally, you want it to “stick to the thing you want it to stick to”, to bounce off and not stick to the proteins you do not want it to stick to. By setting up a physical environment you can test whether the molecule sticks to a given protein or not.
As you can imagine, in our physical world that is laborious, expensive, tricky, and time consuming. Atomwise is able to frame this as a classification problem: where you have a binary classification, does it stick? Yes or No? Atomwise then puts in the structure of the protein and the structure of the molecule to see if they fit together. Having built that predictive system they can screen billions of compounds that are commercially available today and pull down a shortlist of molecules that are worth testing physically.
All in all, Atomwise is predicting if the molecule is going to bind to the protein or not. Through simulation using deep learning AI and machine learning algorithms, they’re saving the actual experiment exercise while offering predictability if the molecule is going to work or not.
Proving the Technology Works to the Pharma Industry
To start with, the life science industry is very skeptical. In some cases, to be a good scientist you have to be skeptical, to look at the data and ask: “how can this data be an artifact?” Atomwise pitch is one that is familiar, in fact has been around for decades, so people are skeptical of their business approach. This creates a burden on Atomwise. In proving their new technology is able to do something never done before, that it actually works. Atomwise must show their product works. They must show this 100 different times on 100 different proteins on different diseases on different parts of biology, in many people’s hands, that it works over and over again without fail.
With that being the goal, Atomwise spun up a program called AIMS – the Artificial Intelligence Molecular Screening Program. Through this program Atomwise is able to solve the problem that has been left open for decades. For example, if you’re a professor at a university and you have been studying a protein, you might have great evidence that if you could block protein XYZ and would have great impact for people with Alzheimer’s, Cancer, or COVID-19. As a professor, you have insights into biology and know how to test whether you’re blocking the protein, but what you need is the drug or the molecule. You need a molecule that can safely and effectively block up protein XYZ. This is where you come to Atomwise and tell them you’re interested in protein XYZ. Atomwise will run the AI and screen billions of molecules to then compile a short list. From there, Atomwise will ship you compounds for you to test, and to share the data back with Atomwise. This procedure has been extremely successful. Over the last year, Atomwise has had over 1,000 applications to come work on this, and an additional 750 projects. Atomwise has the results for over 100 different such projects, so they can actually demonstrate with statistical significance where and how it works. They can look at the success rates and demonstrate over and over again that they can unlock these proteins that otherwise are impossible. And Atomwise can do it faster than setting up a physical experiment. Their partners can do experiments they couldn’t do otherwise, with the ability to ask questions they couldn’t have asked before. The process would be way too hard for them to set up on their own. A huge reason why Atomwise is so valuable.
Advice for Aspiring Entrepreneurs
Do not wait for permission from others to do something, do not wait for the moment from others to say “go”. Always think about what you need to prove, while making sure you’re providing value to other people. You must be providing value to customers, your partners, and collaborators. That’s how proof works. It’s not about doing better on a benchmark, which AI often thinks about, for the benchmarks have to link into people’s lives or business outcomes.
Know you are not going to have all of the right answers. You must iterate your way to success. You must take as many steps and get the gradient updates as quickly as possible, from here you can find progress. If you wait, you do not get back a signal as for what is not working. It’s critically important to keep trying, and to not delay. You learn so much more this way. Take small steps and take many gradient updates.
Future Endeavors
The future is extremely bright for Atomwise. Atomwise recently raised $123 million, Series B, enabling the company to grow and scale in a number of important ways. First, the range of molecules that you can work with today is massive, and it has been becoming more massive in the last 15 years. This is a new world that we’re living in, we’re living through a transition where synthesis on demand lets us do chemistry in a different way, to access chemistry we’ve never been able to access before. The chemical vendors are adding about a billion molecules a month to the catalogs. For context, that’s like taking the entire corporate collection of all of the top 20 pharma companies and putting them together, multiplying it by 10, and adding every month to what you can order out of a catalog today. There’s really no way to test that physically. You have to have incredibly accurate computational approaches to test those molecules to even keep up. Because if you have even a small error rate, the false positives swamp you entirely. Atomwise really has to scale their technology to keep up with the massive amount of chemistry that is out there. They have to scale their technology to work on broader classes of proteins, and to answer questions as they take the molecules deeper into drug discovery.
On the business side, Atomwise is continuing to grow their partnerships, their portfolio to work with Big pharma and Small Pharma. Atomwise has had an increased focus on doing their own discovery. With this kind of scaling, Atomwise gets to grow the team, which is another main focus of the company.
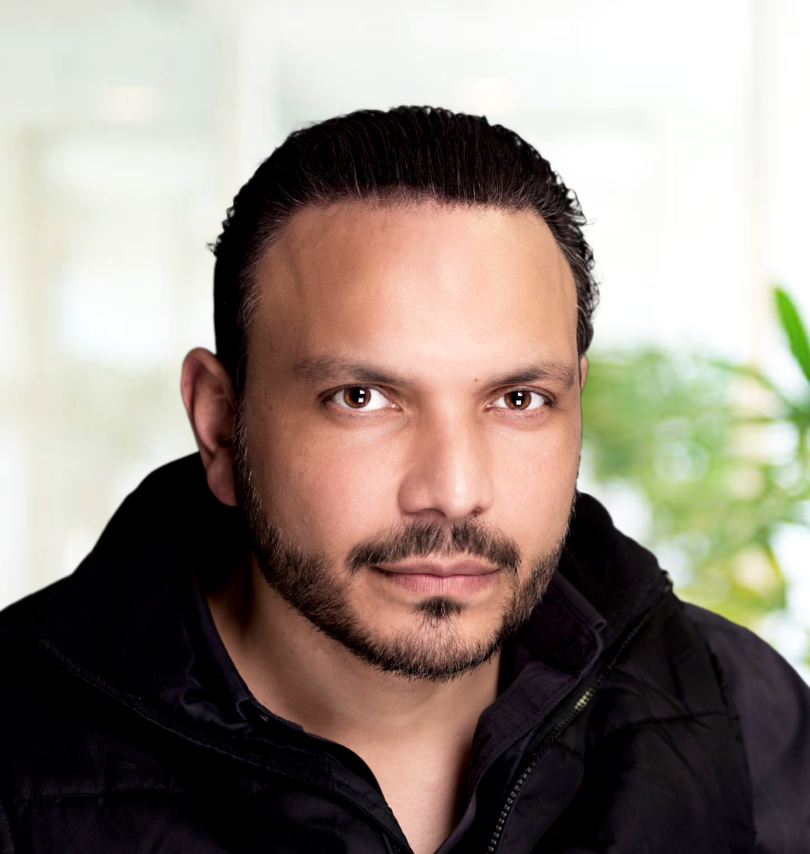
About the Host
Ari Yacobi is a data scientist, a teacher and a storyteller who has spent his career at…Read the Bio